Co-authored by Prof. CHENG Liming and Prof. ZHU Rongrong from the Key Laboratory of Spine and Spinal Cord Injury Repair and Regeneration of the Ministry of Education at the Tongji Hospital of Tongji University, the research paper titled "Deep learning-based predictive identification of neural stem cell differentiation" was published online on May 10th in the internationally renowned journal Nature Communications, and was selected into Editors' Highlights in the area of "Stem
Co-authored by Prof. CHENG Liming and Prof. ZHU Rongrong from the Key Laboratory of Spine and Spinal Cord Injury Repair and Regeneration of the Ministry of Education at the Tongji Hospital of Tongji University, the research paper titled "Deep learning-based predictive identification of neural stem cell differentiation" was published online on May 10th in the internationally renowned journal Nature Communications, and was selected into Editors' Highlights in the area of "Stem Cells" (https://www.nature.com/collections/pkgztbmgly).

Paper published by Prof. CHENG Liming and Prof. ZHU Rongrong in Nature Communications

The paper is titled "Deep learning-based predictive identification of neural stem cell differentiation"
Neural stem cells (NSCs) have the capacity for self-renewal and differentiation, and can be induced into neurons, astrocytes and oligodendrocytes, thus offering important value for regenerative research and application in treating central nervous system (CNS) diseases. The directional differentiation of NSCs into neurons has always been an important research direction in this field, with commonly-used inducers including several neurotrophins, small molecule drugs and hormones. Traditional drug screening and identification methods, such as immunofluorescence, are plagued by problems such as long cycle, high cost and background interference. Therefore, discovering a more efficient method for the predictive differentiation of NSCs is crucial to the research in this field.
This study constructed a NSCs differentiation fate prediction system based on the high-throughput flow cytometry single-cell imaging technology, and verified how well it can predict the fate of NSCs in different scenarios involving various inducers. First, flow cytometry was used to obtain annotated single-cell images of NSCs with different differentiation directions (Figure 1a), and then a convolutional neural network (CNN) was used to extract local image features, forming a well-architected image classification (Figure 1b). The model constructed by this system boasts high general suitability, including neurotrophins and hormones commonly used in laboratories, as well as innovative and cutting-edge nanoparticles. Moreover, this system allows highly accurate and fast prediction within 24 hours through the use of merely bright field single-cell images (Figure 1c).

Figure 1. Workflow of the deep learning-based predictive identification of neural stem cell differentiation
The results show that the prediction system boasts high prediction accuracy in different days and directions of NSC differentiation, and can accurately predict the differentiation direction of NSCs shortly processed with factors, small molecules or nanoparticles on the basis of the single-cell bright-field images of flow cytometry. The prediction of the directions of neurons, astrocytes and oligodendrocytes only took 1 day, 12 hours and 1 day respectively at the shortest time, and the predicted differentiation ratios were 0.827, 0.959 and 0.806 respectively. The prediction results of the independent test set showed that the ratios of cell differentiation were highly consistent with the ratios obtained by immunofluorescence staining experiments (Figure 2a-c). In addition, the results of class activation map (CAM) showed that the system could spontaneously focus on the cells, including the edges and internal organelles (Figure 2d). Compared with traditional molecular experiments and evaluation methods, the proposed system boasts merits such as fast prediction, simple operation, good immunity to interference, forward-looking and high precision, and can overcome many limitations of existing laboratory methods and techniques.

Figure 2. Deep learning-based prediction of the differentiation fate of NSCs under different conditions
ZHU Yanjing and HUANG Ruiqi are the co-first authors of this paper, whilst Prof. CHENG Liming and Prof. ZHU Rongrong are the co-corresponding authors. This study has proposed an advanced tool for the identification of NSC fate with high precision, low false positive rate, fast speed, wide applicability, simple operation, and good cost-effectiveness. This could greatly streamline and speed up the identification of new induction methods for NSCs differentiation, and boasts high application value for the research into the stem cell therapy of CNS.
Link: https://www.nature.com/articles/s41467-021-22758-0
https://news.tongji.edu.cn/info/1003/77637.htm
Cells" (https://www.nature.com/collections/pkgztbmgly). 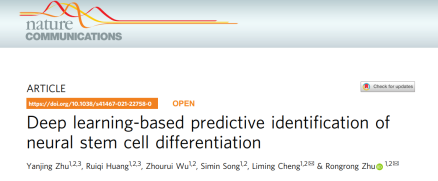
Paper published by Prof. CHENG Liming and Prof. ZHU Rongrong in Nature Communications
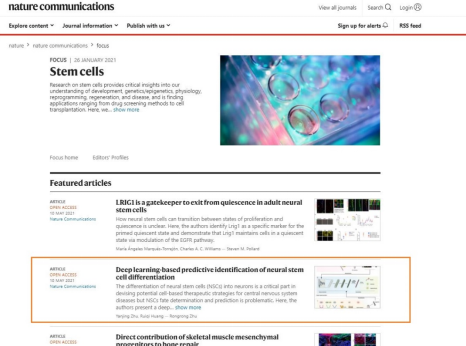
The paper is titled "Deep learning-based predictive identification of neural stem cell differentiation"
Neural stem cells (NSCs) have the capacity for self-renewal and differentiation, and can be induced into neurons, astrocytes and oligodendrocytes, thus offering important value for regenerative research and application in treating central nervous system (CNS) diseases. The directional differentiation of NSCs into neurons has always been an important research direction in this field, with commonly-used inducers including several neurotrophins, small molecule drugs and hormones. Traditional drug screening and identification methods, such as immunofluorescence, are plagued by problems such as long cycle, high cost and background interference. Therefore, discovering a more efficient method for the predictive differentiation of NSCs is crucial to the research in this field.
This study constructed a NSCs differentiation fate prediction system based on the high-throughput flow cytometry single-cell imaging technology, and verified how well it can predict the fate of NSCs in different scenarios involving various inducers. First, flow cytometry was used to obtain annotated single-cell images of NSCs with different differentiation directions (Figure 1a), and then a convolutional neural network (CNN) was used to extract local image features, forming a well-architected image classification (Figure 1b). The model constructed by this system boasts high general suitability, including neurotrophins and hormones commonly used in laboratories, as well as innovative and cutting-edge nanoparticles. Moreover, this system allows highly accurate and fast prediction within 24 hours through the use of merely bright field single-cell images (Figure 1c).
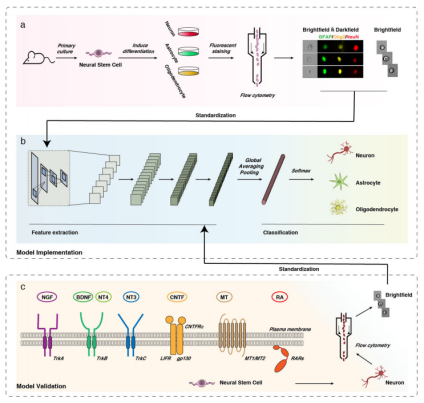
Figure 1. Workflow of the deep learning-based predictive identification of neural stem cell differentiation
The results show that the prediction system boasts high prediction accuracy in different days and directions of NSC differentiation, and can accurately predict the differentiation direction of NSCs shortly processed with factors, small molecules or nanoparticles on the basis of the single-cell bright-field images of flow cytometry. The prediction of the directions of neurons, astrocytes and oligodendrocytes only took 1 day, 12 hours and 1 day respectively at the shortest time, and the predicted differentiation ratios were 0.827, 0.959 and 0.806 respectively. The prediction results of the independent test set showed that the ratios of cell differentiation were highly consistent with the ratios obtained by immunofluorescence staining experiments (Figure 2a-c). In addition, the results of class activation map (CAM) showed that the system could spontaneously focus on the cells, including the edges and internal organelles (Figure 2d). Compared with traditional molecular experiments and evaluation methods, the proposed system boasts merits such as fast prediction, simple operation, good immunity to interference, forward-looking and high precision, and can overcome many limitations of existing laboratory methods and techniques.
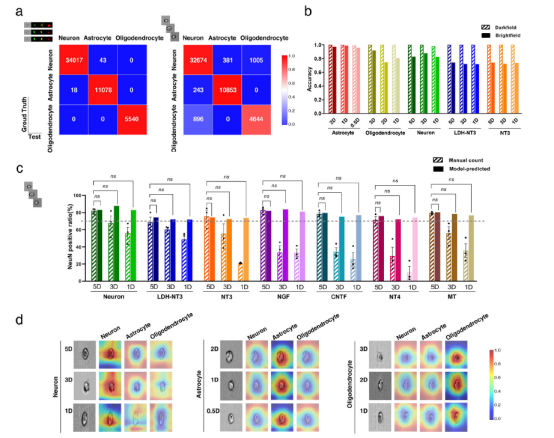
Figure 2. Deep learning-based prediction of the differentiation fate of NSCs under different conditions
ZHU Yanjing and HUANG Ruiqi are the co-first authors of this paper, whilst Prof. CHENG Liming and Prof. ZHU Rongrong are the co-corresponding authors. This study has proposed an advanced tool for the identification of NSC fate with high precision, low false positive rate, fast speed, wide applicability, simple operation, and good cost-effectiveness. This could greatly streamline and speed up the identification of new induction methods for NSCs differentiation, and boasts high application value for the research into the stem cell therapy of CNS.
Link: https://www.nature.com/articles/s41467-021-22758-0
https://news.tongji.edu.cn/info/1003/77637.htm