Recently, CHEN Longping, an undergraduate student of the Year 2019 from the School of Automotive Studies, published a research paper entitled "Triboelectric nanogenerator sensors for intelligent steering wheel aiming at automated driving" as the first author in the academic journal Nano Energy. This paper is an achievement of Tongji University's undergraduate innovation project, representing the interdisciplinary integration of vehicle engineering, intelligent science and technology, and cooperation among universities, enterprises, and industry in education, research, and production. His supervisors, Professor CHEN Hong, and Professor HUANG Yanjun, are the paper's co-corresponding authors.
In recent years, advances in artificial intelligence, sensing methods, communication technology, and control theory have accelerated the development of autonomous vehicles. Human Machine Interaction System (HMI) is an important component of intelligent vehicles and an important link between people and vehicles. The presence of HMI can enhance the driving experience while monitoring driver behavior. Sensors play a crucial role in obtaining information about the environment and the driver for analysis of the driving status. However, the stability, accuracy, and power requirements of cameras and wearable devices have limited the development of traditional sensing devices. Triboelectric nanosensors (TENGs) came into being for their self-powered high sensitivity and small size. The research proposes using TENG as the primary interface for human-machine interaction - the steering wheel- to provide a compact, efficient, and self-powered solution for the HMI system in autonomous vehicles. This research is based on such projects as "Safe and Reliable Human Machine Driving System in an Open Environment" under the major project "New Generation Artificial Intelligence" funded by the Ministry of Science and Technology Innovation 2030.
The overall framework of this study is shown in the figure below. The self-designed TENG is set on the steering wheel rim to collect the driver's grip signal, which is processed by a microcontroller and sent to the upper computer for analysis. The experimental results have shown that due to the high sensitivity of the sensor and the physical features of the grip force change ahead of the angle change, TENG's response time is on average 0.55 seconds ahead of traditional shaft angle sensors (SAS). It is likely to use this time for driver psychological prediction, which provides a new approach for vehicle avoidance and collaborative human-machine control. The study used grip information for steering recognition, compared multiple classification algorithms, and proved that Support Vector Machine (SVM) has a high classification accuracy of up to 97.22%. This will be helpful in making traffic accident analyses and personalized collaborative driving strategies. A case of a cooperative steering control scheme using Model predictive control (MPC) and TENG is introduced, which compares the response time of driver-independent control and human-machine cooperative control in lane-changing scenarios. The experimental results show that the average response time of cooperative control is 0.78s ahead of that of independent control, which is of great significance for improving the handling safety of vehicles at high speed.
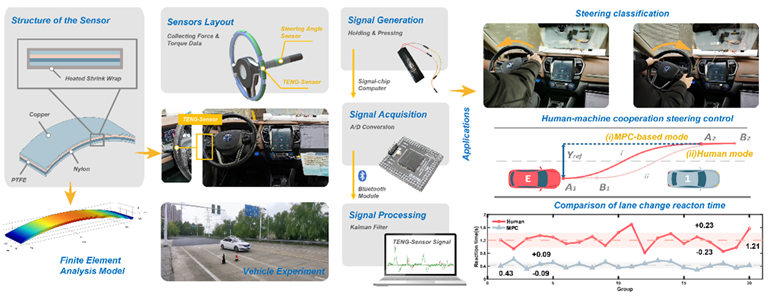
A Safe and Reliable Human Machine Driving System in an Open Environment
The student members of the project team include CHEN Shiyang, an undergraduate student of the Year 2018 from the School of Automotive Studies, CHEN Longping, YU Ninghai, and MO Jingyue, all undergraduate students of the Year 2019. The team completed a series of research processes, including field research, sensor preparation, model simulation, hardware design, algorithm development, experiments, and paper writing, and achieved fruitful results.
Paper link: https://doi.org/10.1016/j.stem.2018.04.013