On March 25, a research paper entitled "Autonomous information of complex network dynamics from incomplete and noisy data" was published online on Nature · Computational Science. The research was completed by Professor YAN Gang from the School of Physical Science and Engineering of Tongji University and Shanghai Autonomous Intelligent Unmanned System Science Center. In this research, the authors propose a two-phase approach to the autonomous interference in data-driven complex network, which realizes the robust interference in incompleteness and noises. This approach provides an important algorithm for the automatic and intelligent discovery of the underlying principles of real complex networks. It is worth mentioning that this algorithm can effectively verify the early spreading of SARS and COVID-19.
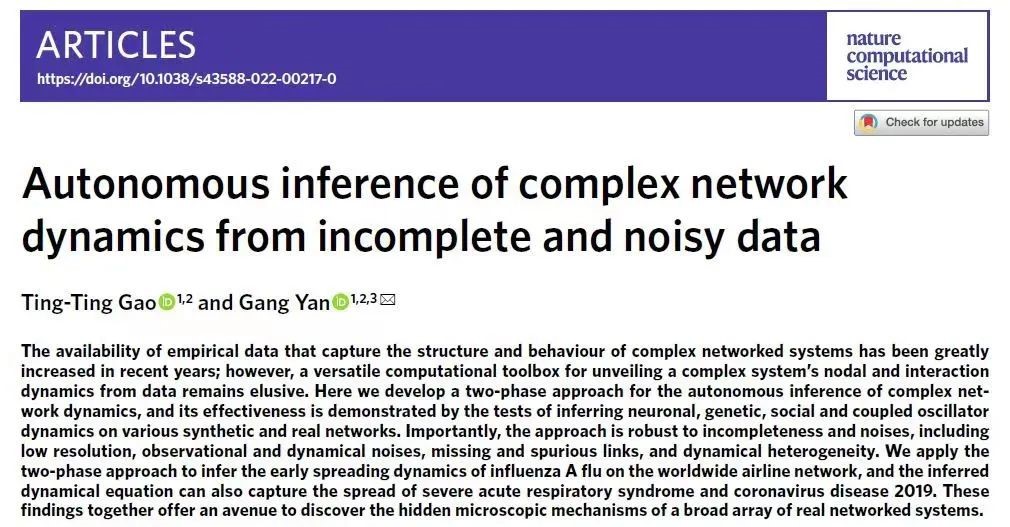
Paper published on Nature Computational Science
A complex system is a non-trivial system formed by the interaction of a large number of nodes, such as unmanned cluster system, Internet of things, neural network and social network. With the continuous development of digital and micro experimental technology, a large number of observation data about practical complex systems have been accumulated, including network structure and node activity sequence. Finding the underlying principle from data is a process that research often needs to go through. Nowadays, with the vigorous rise of intelligence, whether we can use artificial intelligence to accelerate the process of scientific discovery, such as automatically inferring the dynamic equations that determine the behavior of complex systems from data, is an issue of common concern in academia and industry. Previous studies focused on the interference of dynamic equations of a single node or a small number of nodes. It is more challenging for complex networked systems with a large number of nodes. For example, not only the node activity data contains noise, but also the network structure data may be inaccurate. The node dynamics may be heterogeneous, and the macro behavior of the system may mask the micro mechanism of dynamic coupling between nodes. In face the challenge, two new approaches are proposed. The algorithm can infer the hidden network dynamic equations from the observation data efficiently and accurately, and provides an effective new way to discover the underlying principles of complex systems automatically.
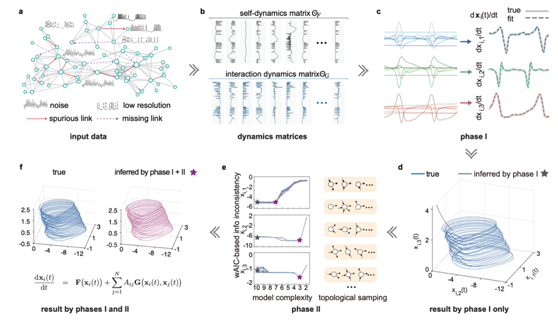
Figure 1. Overview of autonomous interference algorithms for complex networked systems. The observed network and node data (a) are mapped to the time-varying basic function matrix (b), the most relevant basic functions are identified through the regression of the overall perspective and form the model subspace (c), then the optimal function combination and coefficient (E) are inferred through the topological sampling of the local perspective and the criterion of information theory, and finally the system dynamic equation (f) with concise form and generative power.
The algorithm with strong robustness can resist the uncertainty of missing edge, pseudo connected edge, observation noise, correlation noise and intrinsic randomness in the observation data, and can still realize interference when the macro behavior synchronization is strong. Compared with the previous intelligent interference for single or a small number of nodes, the approach proposed in this study can search in more complex and non-orthogonal term space, and performs better in the five model system tests for data uncertainty. The framework is also applied to the inference of the global pandemic dynamics. Fig. 2, based on the H1N1 spreading data, a simple ordinary differential equation for describing the early spreading process is found. The equation can simultaneously depict the early spreading behavior of SARS and COVID-19, and verify the effectiveness and universality of the approach.
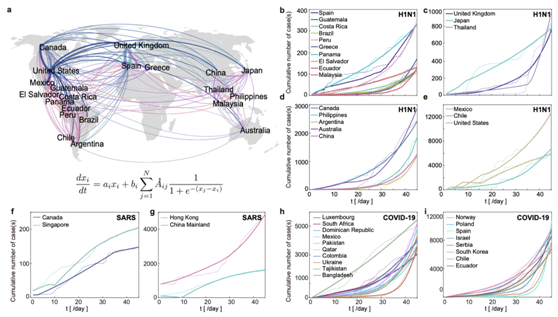
Figure 2. Transmission dynamics interference of global infectious diseases. Based on the global aviation network (a) and regional H1N1 infection data, the early diffusion equation is inferred. The evolution of the equation is consistent with the observed data (B-E) and can be used to characterize the early diffusion behavior of SARS and COVID-19 (f-I).
Professor YAN Gang is the corresponding author of the paper, and doctoral student GAO Tingting is the first author of the paper. The research was supported by National Natural Science Foundation of China, National Science and Technology Innovation 2030, and Shanghai Municipal Science and Technology.
Link to paper:https://www.nature.com/articles/s43588-022-00217-0
Source: https://news.tongji.edu.cn/info/1003/80382.htm